What Is Cross-Validation?
Cross-validation is a method used in chemistry and a wide range of other scientific fields to compare the results of multiple experimental methods with the same goal. Ideally, cross-validation will validate both experimental methods by returning the same results. Different results may indicate human error or errors in experimental design. The differences can be used to identify errors and to refine one or more of the experimental methods until consistent and repeatable results are obtained.
For cross-validation to be successful, it is generally necessary for researchers to know that one of the methods returns accurate results. The goal, then, is to make the new and unconfirmed method, or the comparator, return results identical to those of the known method, or the reference. If neither method is known to be accurate, they can probably be adjusted to return the same results, but there is still no guarantee that those results are correct.
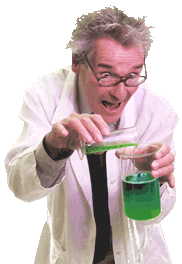
Researchers often use cross-validation when introducing a new, more efficient experimental method that is intended to replace an older method. The new method is only useful if it can be used for the same purpose as the method it is intended to replace. Cross-validation is used to ensure that the new method is just as effective as the old and that efficiency does not come at the price of accuracy.
The results of experiments used for cross-validation can be prepared qualitatively or quantitatively based on the nature of the experiment. The success of some simple chemistry experiments can be assessed through simple visual cues such as color change. A new method that results in the same color change can be, in some cases,judged successful. Most modern scientific research is, however, largely based on quantitative methods. As such, quantitative information must be compared, and differences in numerical data are used to judge the success or failure of a validation experiment.
Much cross-validation result relies on large bodies of statistical data rather than on qualitative information or on one or two values such as temperature or acidity. For such statistical data, there is no single specific number or set of numbers that is correct while all others are incorrect. The success of a cross-validation is judged based on whether or not the data returned falls within a certain threshold of acceptable error. In such experiments, some of the values returned may be acceptable while others are erroneous, indicating that particular parts of the tested methods must be revised.
AS FEATURED ON:
AS FEATURED ON:


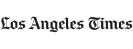

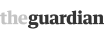
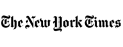
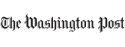


Discuss this Article
Post your comments